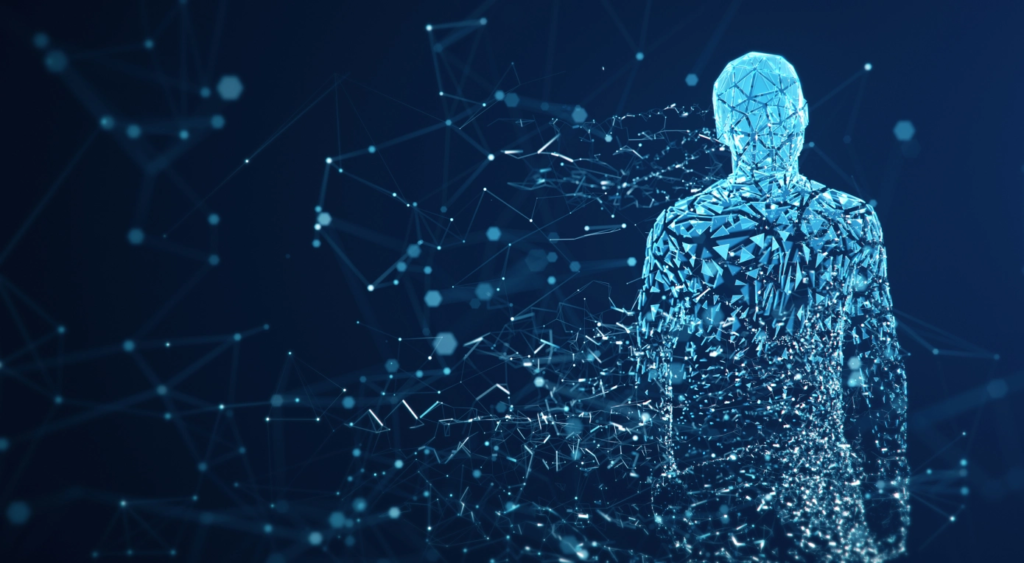
The field of image generation is constantly evolving, with new techniques and models being developed at a rapid pace. While diffusion models like those used in popular tools such as Midjourney and Stable Diffusion have been impressive, OpenAI’s latest research on “consistency models” shows promising results in terms of speed and efficiency.
Consistency models are a different approach compared to diffusion models. In diffusion, the model gradually removes noise from an initial image made entirely of noise, iteratively moving it closer to the target prompt. While this has led to impressive results, it requires multiple steps and can be computationally expensive and slow for real-time applications.
The goal of consistency models is to achieve good results in just one or at most two computation steps. These models are trained, similar to diffusion models, to observe the process of image destruction. However, instead of requiring multiple steps, consistency models are designed to take an image at any level of obscuration, whether it has little or a lot of missing information, and generate a complete source image in just one step.
OpenAI’s recent research on consistency models has been published as a preprint, and while it may be technical and not accompanied by significant fanfare, the results are noteworthy. These experimental models show potential in significantly reducing the computational cost and time required for image generation, which could have practical applications in real-time scenarios. However, as with any research, further development, testing, and refinement will be needed before widespread adoption in practical applications.
But I hasten to add that this is only the most hand-wavy description of what’s happening. It’s this kind of paper:
The generated images from the consistency models may not be visually stunning, with some images even being considered mediocre. However, the key advancement is that these images are generated in just one or a few steps, as opposed to the hundreds or thousands of steps required by diffusion models. Additionally, the consistency models are capable of generalizing to various tasks such as colorization, upscaling, sketch interpretation, infilling, and more, also achievable within a single step, although sometimes improved with a second step. This demonstrates the potential of consistency models in significantly reducing the computational overhead and time required for a wide range of image generation tasks.
The significance of consistency models lies in their potential to break the self-limiting cycle of traditional machine learning research. Typically, a technique is established, improved upon, and tuned over time with increased computational resources to produce better results. However, this process has limitations in terms of the amount of computation that can be dedicated to a given task.
Consistency models represent a shift towards more efficient techniques that may initially produce inferior results but are highly efficient in terms of computation. This indicates that OpenAI, a leading AI research organization, is actively looking beyond diffusion models towards next-generation use cases.
Diffusion models may be suitable for tasks that require a large number of iterations and significant computational resources. However, consistency models may be better suited for scenarios where efficiency is crucial, such as running image generators on mobile devices without draining the battery or providing quick results in live chat interfaces. OpenAI’s researchers, including renowned experts like Ilya Sutskever, are actively exploring the potential of consistency models alongside other contributors such as Yang Song, Prafulla Dhariwal, and Mark Chen.
The future of AI research is likely to be multimodal and multi-model, and the role of consistency models in OpenAI’s arsenal of techniques will depend on how the research progresses.